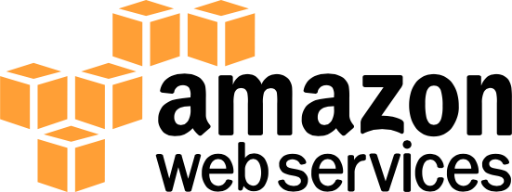
S3 Archiving for LogScale Cloud
Security Requirements and Controls
Change S3 archiving settings
permission
LogScale supports archiving ingested logs to Amazon S3. The archived logs are then available for further processing in any external system that integrates with S3. The files written by LogScale in this format are not searchable by LogScale — this is an export meant for other systems to consume.
When S3 Archiving is enabled all the events in repository are backfilled into S3 and then it archives new events by running a periodic job inside all LogScale nodes, which looks for new, unarchived segment files. The segment files are read from disk, streamed to an S3 bucket, and marked as archived in LogScale.
An admin user needs to set up archiving per repository. After selecting a repository on the LogScale front page, the configuration page is available under Settings.
Note
For slow-moving datasources it can take some time before segment files are completed on disk and then made available for the archiving job. In the worst case, before a segment file is completed, it must contain a gigabyte of uncompressed data or 30 minutes must have passed. The exact thresholds are those configured as the limits on mini segments.
Important
S3 archiving is not supported for S3 buckets where object locking is enabled.
For more information on segments files and datasources, see Ingest and LogScale Internal Architecture
LogScale Cloud Setup
Enabling LogScale Cloud to write to your S3 bucket means setting up AWS cross-account access.
In AWS:
Log in to the AWS console and navigate to your S3 service page.
Click the name of the bucket where archived logs should be written.
Note
Please follow the instructions on the AWS docs on naming conventions. In particular, using dashes not periods as a separator, and ensuring you do not repeat dashes and dots.
Click
Scroll down to the Access Control Lists and click
Scroll down and click the
buttonEnter the canonical ID for LogScale:
logscalef2631ff87719416ac74f8d9d9a88b3e3b67dc4e7b1108902199dea13da892780
Additionally give it Write on the objects.
In LogScale:
Go to the repository you want to archive and select
→ .Configure by giving the bucket name, region, and then
.
S3 Archived Log Re-ingestion
To re-ingest log data that has been written to an S3 bucket through S3 archiving can be achieved by using LogScale Collector and the native JSON parsing within LogScale.
This process has the following requirements:
The files will need to be downloaded from the S3 bucket to the machine running the LogScale Collector. The S3 files cannot be accessed natively by the log collector.
The ingested events will be ingested into the repository that is created for the purpose of receiving the data.
To re-ingest logs this way:
Create a repo in LogScale where the ingested data will be stored. See Creating a Repository or View.
Create an ingest token, and choose the JSON parser. See Assigning Parsers to Ingest Tokens.
Install the Falcon LogScale Collector to read from a file using the
.gz
extension as the file match. For example, using a configuration similar to this:yaml#dataDirectory is only required in the case of local configurations and must not be used for remote configurations files. dataDirectory: data sources: bucketdata: type: file # Glob patterns include: - /bucketdata/*.gz sink: my_humio_instance parser: json ...
For more information, see Sources & Examples.
Copy the log file from the S3 bucket into the configured directory (
/bucketdata
in the above example.
The Log Collector will read the file that has been copied, send it to LogScale, where the JSON event data will be parsed and recreated.
S3 Storage Format and Layout
When uploading a segment file, LogScale creates the S3 object key based on the tags, start date, and repository name of the segment file. The resulting object key makes the archived data browsable through the S3 management console.
LogScale uses the following pattern:
REPOSITORY/TYPE/TAG_KEY_1/TAG_VALUE_1/../TAG_KEY_N/TAG_VALUE_N/YEAR/MONTH/DAY/START_TIME-SEGMENT_ID.gz
Where:
REPOSITORY
Name of the repository
type
Keyword (static) to identify the format of the enclosed data.
TAG_KEY_1
Name of the tag key (typically the name of parser used to ingest the data, from the #type field)
TAG_VALUE
Value of the corresponding tag key.
YEAR
Year of the timestamp of the events
MONTH
Month of the timestamp of the events
DAY
Day of the timestamp of the events
START_TIME
The start time of the segment, in the format
HH-MM-SS
SEGMENT_ID
The unique segment ID of the event data
An example of this layout can be seen in the file list below:
$ s3cmd ls -r s3://logscale2/accesslog/
2023-06-07 08:03 1453 s3://logscale2/accesslog/type/kv/2023/05/02/14-35-52-gy60POKpoe0yYa0zKTAP0o6x.gz
2023-06-07 08:03 373268 s3://logscale2/accesslog/type/kv/humioBackfill/0/2023/03/07/15-09-41-gJ0VFhx2CGlXSYYqSEuBmAx1.gz
Read more about Event Tags.
File Format
LogScale supports two formats for storage, native format and NDJSON.
Native Format
The native format is the raw data, i.e. the equivalent of the @rawstring of the ingested data:
accesslog127.0.0.1 - - [07/Mar/2023:15:09:42 +0000] "GET /falcon-logscale/css-images/176f8f5bd5f02b3abfcf894955d7e919.woff2 HTTP/1.1" 200 15736 "http://localhost:81/falcon-logscale/theme.css" "Mozilla/5.0 (Macintosh; Intel Mac OS X 10_15_7) AppleWebKit/537.36 (KHTML, like Gecko) Chrome/110.0.0.0 Safari/537.36" 127.0.0.1 - - [07/Mar/2023:15:09:43 +0000] "GET /falcon-logscale/css-images/alert-octagon.svg HTTP/1.1" 200 416 "http://localhost:81/falcon-logscale/theme.css" "Mozilla/5.0 (Macintosh; Intel Mac OS X 10_15_7) AppleWebKit/537.36 (KHTML, like Gecko) Chrome/110.0.0.0 Safari/537.36" 127.0.0.1 - - [09/Mar/2023:14:16:56 +0000] "GET /theme-home.css HTTP/1.1" 200 70699 "http://localhost:81/" "Mozilla/5.0 (Macintosh; Intel Mac OS X 10_15_7) AppleWebKit/537.36 (KHTML, like Gecko) Chrome/111.0.0.0 Safari/537.36" 127.0.0.1 - - [09/Mar/2023:14:16:59 +0000] "GET /css-images/help-circle-white.svg HTTP/1.1" 200 358 "http://localhost:81/" "Mozilla/5.0 (Macintosh; Intel Mac OS X 10_15_7) AppleWebKit/537.36 (KHTML, like Gecko) Chrome/111.0.0.0 Safari/537.36" 127.0.0.1 - - [09/Mar/2023:14:16:59 +0000] "GET /css-images/logo-white.svg HTTP/1.1" 200 2275 "http://localhost:81/" "Mozilla/5.0 (Macintosh; Intel Mac OS X 10_15_7) AppleWebKit/537.36 (KHTML, like Gecko) Chrome/111.0.0.0 Safari/537.36"
NDJSON Format
The default archiving format is NDJSON When using NDJSON, the parsed fields will be available along with the raw log line. This incurs some extra storage cost compared to using raw log lines but gives the benefit of ease of use when processing the logs in an external system.
json{"#type":"default","url":"/data-analysis-1.100/js/ini.min.js","userid":"-","statuscode":"200","client":"192.168.1.108","@timezone":"+01:00","@timestamp.nanos":"0","@rawstring":"192.168.1.108 - - [03/07/2024:04:45:19 +0100] \"GET /data-analysis-1.100/js/ini.min.js HTTP/1.1\" 200 1044","@id":"MqHKxw2QoBPZyNqbJRRs4ECC_0_0_1719978319","method":"GET","@timestamp":1719978319000,"@ingesttimestamp":"1719981913077","responsesize":"1044","httpversion":"HTTP/1.1"} {"#type":"default","url":"/data-analysis-1.100/css-images/linkedin.svg","userid":"-","statuscode":"200","client":"192.168.1.168","@timezone":"+01:00","@timestamp.nanos":"0","@rawstring":"192.168.1.168 - - [03/07/2024:04:45:40 +0100] \"GET /data-analysis-1.100/css-images/linkedin.svg HTTP/1.1\" 200 400","@id":"MqHKxw2QoBPZyNqbJRRs4ECC_0_1_1719978340","method":"GET","@timestamp":1719978340000,"@ingesttimestamp":"1719981926972","responsesize":"400","httpversion":"HTTP/1.1"} {"#type":"default","url":"/logscale-repo-schema/logscale-repo-schema-humio-fleet.html","userid":"-","statuscode":"200","client":"192.168.1.14","@timezone":"+01:00","@timestamp.nanos":"0","@rawstring":"192.168.1.14 - - [03/07/2024:04:45:40 +0100] \"GET /logscale-repo-schema/logscale-repo-schema-humio-fleet.html HTTP/1.1\" 200 48804","@id":"MqHKxw2QoBPZyNqbJRRs4ECC_0_2_1719978340","method":"GET","@timestamp":1719978340000,"@ingesttimestamp":"1719981926990","responsesize":"48804","httpversion":"HTTP/1.1"} {"#type":"default","url":"/data-analysis/js/theme.js","userid":"-","statuscode":"200","client":"192.168.1.109","@timezone":"+01:00","@timestamp.nanos":"0","@rawstring":"192.168.1.109 - - [03/07/2024:04:45:40 +0100] \"GET /data-analysis/js/theme.js HTTP/1.1\" 200 14845","@id":"MqHKxw2QoBPZyNqbJRRs4ECC_0_3_1719978340","method":"GET","@timestamp":1719978340000,"@ingesttimestamp":"1719981927004","responsesize":"14845","httpversion":"HTTP/1.1"} {"#type":"default","url":"/falcon-logscale-cloud/js/jquery.js","userid":"-","statuscode":"200","client":"192.168.1.57","@timezone":"+01:00","@timestamp.nanos":"0","@rawstring":"192.168.1.57 - - [03/07/2024:04:45:40 +0100] \"GET /falcon-logscale-cloud/js/jquery.js HTTP/1.1\" 200 89947","@id":"MqHKxw2QoBPZyNqbJRRs4ECC_0_4_1719978340","method":"GET","@timestamp":1719978340000,"@ingesttimestamp":"1719981927023","responsesize":"89947","httpversion":"HTTP/1.1"}
A single NDJSON line is just a JSON object, which formatted looks like this:
json{ "#type" : "default", "@id" : "MqHKxw2QoBPZyNqbJRRs4ECC_0_0_1719978319", "@ingesttimestamp" : "1719981913077", "@rawstring" : "192.168.1.108 - - [03/07/2024:04:45:19 +0100] \"GET /data-analysis-1.100/js/ini.min.js HTTP/1.1\" 200 1044", "@timestamp" : 1719978319000, "@timestamp.nanos" : "0", "@timezone" : "+01:00", "client" : "192.168.1.108", "httpversion" : "HTTP/1.1", "method" : "GET", "responsesize" : "1044", "statuscode" : "200", "url" : "/data-analysis-1.100/js/ini.min.js", "userid" : "-" }
Each record will include the full detail for each event, including parsed fields, the original raw event string, and tagged field entries.
Tag Grouping
If tag grouping is defined for a repository, the segment files will be split by each unique combination of tags present in a file. This results in a file in S3 per each unique combination of tags. The same layout pattern is used as in the normal case. The reason for doing this is to make it easier for a human operator to determine whether a log file is relevant.